The Power of AI in Edge Computing: Revolutionizing Real-Time Data Processing
Explore the ways in which AI in edge computing is changing various industries through real-time data processing, improved performance, and quicker, more intelligent decision-making. Find out about the main advantages, practical applications, and future developments of AI at the edge.
AI AND TECHNOLOGY
9/19/20244 min read
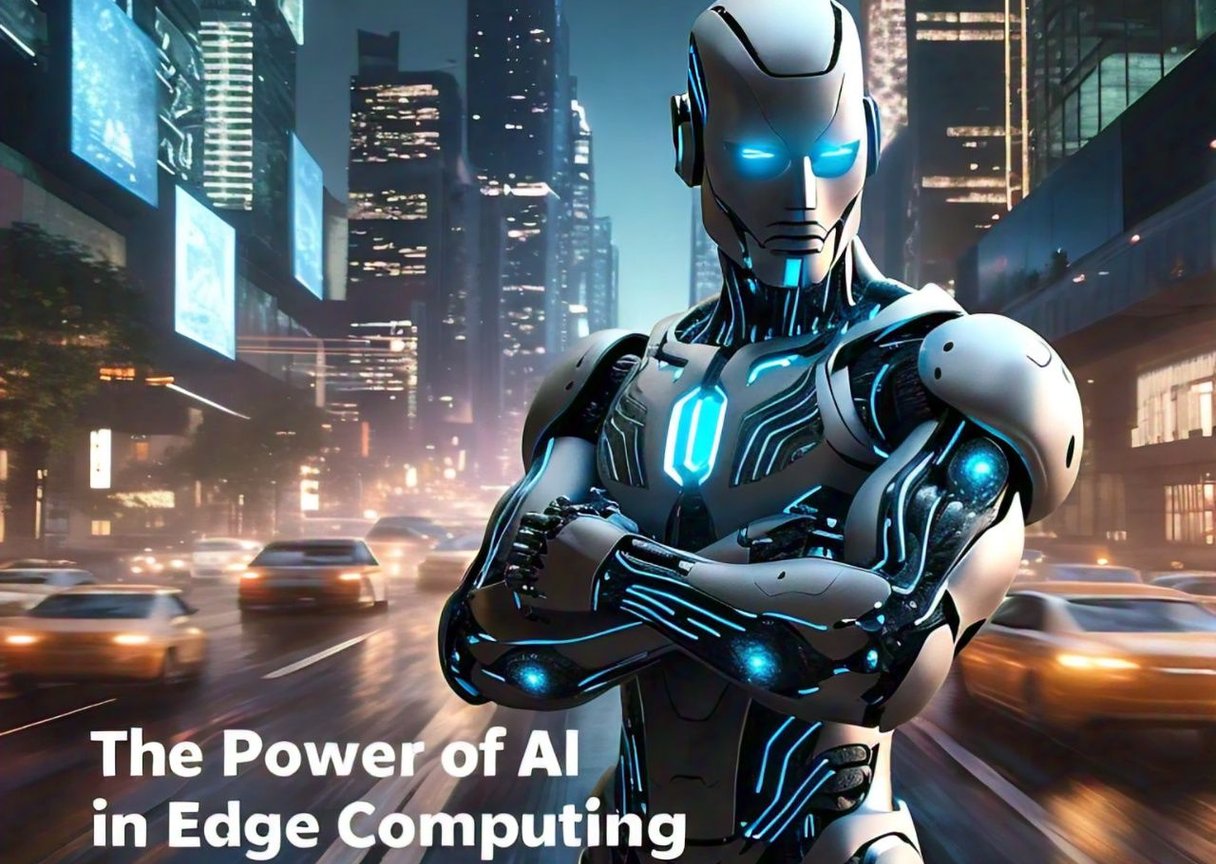
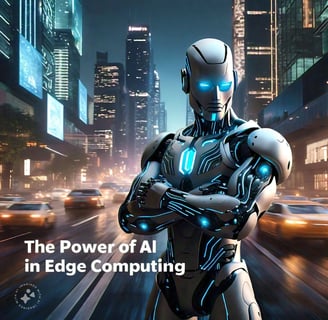
As industries increasingly adopt AI and Internet of Things (IoT) technologies, edge computing has became a key innovation, allowing real-time data processing closer to its origin. By integrating AI with edge computing, organizations can achieve quicker decision-making, reduced latency, and improved data security. In this blog post, we will dig into the revolutionary effects of AI in edge computing, key applications across various industries, and the benefits and challenges associated with deploying AI at the edge.
What is Edge Computing?
Edge computing is the practice of processing data at or near its source, instead of relying solely on centralized cloud servers. This localized processing decreases both the time and bandwidth required to send data to remote servers, resulting in quicker responses and enhanced performance.
When AI is used in edge computing, it enables devices to carry out complex analyses and make decisions without needing to connect to distant data centers. AI algorithms operate directly on edge devices, such as sensors, cameras, smartphones, or IoT devices, leading to real-time, actionable insights.
Benefits of AI in Edge Computing
Low Latency and Real-Time Decision-Making
A major advantage of combining AI with edge computing is the major reduction in latency. Since data is processed locally, AI models can make decisions in real time without requiring data to travel to and from cloud servers. This is especially critical for time-sensitive applications like autonomous vehicles, robotics, and healthcare, where split-second decisions can be critical.
Enhanced Data Privacy and Security
Edge computing provides improved control over sensitive information. By processing data locally on devices, it reduces the volume of information sent to the cloud, which minimizes the risk of data breaches and cyberattacks. Sectors such as finance, healthcare, and retail can use AI-driven edge solutions to safeguard user privacy while still achieving valuable insights.
Reduced Bandwidth and Cloud Dependency
By applying AI at the edge, reliance on cloud resources is minimized, resulting in more efficient bandwidth usage. With millions of connected IoT devices producing vast amounts of data, the continuous transmission of this information to the cloud for processing can lead to congestion and higher costs. AI in edge computing enables local data filtering and analysis, allowing only pertinent insights to be forwarded to the cloud, which conserves bandwidth and diminishes cloud dependency.
Scalability and Flexibility
Implementing AI algorithms on edge devices enhances scalability across distributed systems. Rather than sending every data point to the cloud, companies can deploy AI models on-site, which is particularly advantageous in industries with extensive IoT deployments, such as agriculture, smart cities, and manufacturing.
Principal Uses of AI in Edge Computing
Autonomous Vehicles
Self-driving cars depend on real-time data processing to make swift decisions, including identifying obstacles, recognizing traffic signals, and determining safe driving routes. AI positioned at the edge enables these vehicles to process sensor data instantly, guaranteeing safe and efficient operation without needing cloud connectivity, which could result in delays.
Healthcare and Remote Monitoring
The integration of edge computing with AI is transforming remote healthcare monitoring. Wearable devices that incorporate AI algorithms can examine critical signs, identify anomalies, and alert healthcare professionals in real time. This minimizes the necessity for constant data transmission to the cloud and allows for fast interventions when required, eventually enhancing patient outcomes.
Smart Manufacturing
In smart factories, AI-driven edge computing is employed to oversee machinery, enhance production lines, and foresee maintenance requirements before failures happen. By analyzing data on-site, manufacturers can reduce downtime, boost productivity, and address issues more swiftly. Edge AI also contributes to quality control by using computer vision to identify defects in real time.
Retail and Customer Experience
AI at the edge is improving retail operations by assisting intelligent inventory management, analyzing customer behavior, and creating personalized shopping experiences. For instance, in smart stores, AI-enabled cameras can observe customer movements and preferences, providing targeted recommendations instantly. Edge computing guarantees that these insights are delivered without delay, eliminating needing cloud processing.
Smart Cities and IoT
In smart cities, AI-enabled edge devices are used to control traffic flow, track air quality, and optimize energy use. By processing data locally, these systems can make immediate modifications to enhance urban living conditions. For example, traffic lights integrated with AI algorithms can adjust their timing dynamically based on real-time traffic data, helping to alleviate congestion.
Challenges of AI in Edge Computing
Although the advantages of AI in edge computing are major, several challenges must be addressed:
Computational Power
Edge devices, including sensors and cameras, often possess limited processing capability when compared to centralized cloud servers. Running AI algorithms on these devices can be computationally intensive, and adjusting these models for edge devices necessitates considerable technical knowledge.
Model Accuracy vs. Latency
To ensure low-latency decision-making, AI models deployed on edge devices are frequently less complex than their cloud-based equivalents. Striking a balance between model accuracy and processing speed presents a challenge, especially in fields like healthcare or autonomous driving, where precision is essential.
Deployment and Maintenance
Implementing AI models on distributed edge devices can be more complicated than deploying them in the cloud. Edge environments differ greatly, and guaranteeing that AI models operate consistently across various devices and networks can be difficult. Frequent updates and maintenance of edge AI systems are also essential for maintaining performance and security.
The Future of AI in Edge Computing
The partnership of AI and edge computing is set to inspire further advancements across various industries. Several important trends are shaping the future of AI at the edge:
5G and Edge AI
The introduction of 5G networks will promote the uptake of AI at the edge by offering quicker, more reliable connectivity for IoT devices. With 5G, AI-powered edge systems will possess even greater capabilities for real-time data processing, making them suitable for applications such as smart cities, industrial automation, and autonomous vehicles.
AI Model Optimization
As edge AI becomes increasingly widespread, researchers and developers are concentrating on optimizing AI models for smaller, more energy-efficient edge devices. Techniques like model pruning and quantization are enabling the deployment of strong AI algorithms on hardware-constrained devices without compromising accuracy or performance.
Edge AI in the Consumer Market
AI-powered edge devices, including smart home systems and wearable technology, are anticipated to gain popularity among consumers. These devices will provide enhanced personalization, real-time decision-making, and improved user privacy, with AI algorithms performing data processing on-site rather than in the cloud.
Explore
Motivating material related to health, technology, finance, and lifestyle in everyday life.
Connect
Discover
info@webslike.com
© 2024. All rights reserved.