Understanding Explainable AI (XAI): Making AI Decisions Transparent and Accountable
Explore the concept of Explainable AI (XAI) and its role in improving transparency, accountability, and trust in AI decision-making processes. Discover the main advantages, challenges, and future developments related to XAI.
AI AND TECHNOLOGY
9/19/20244 min read
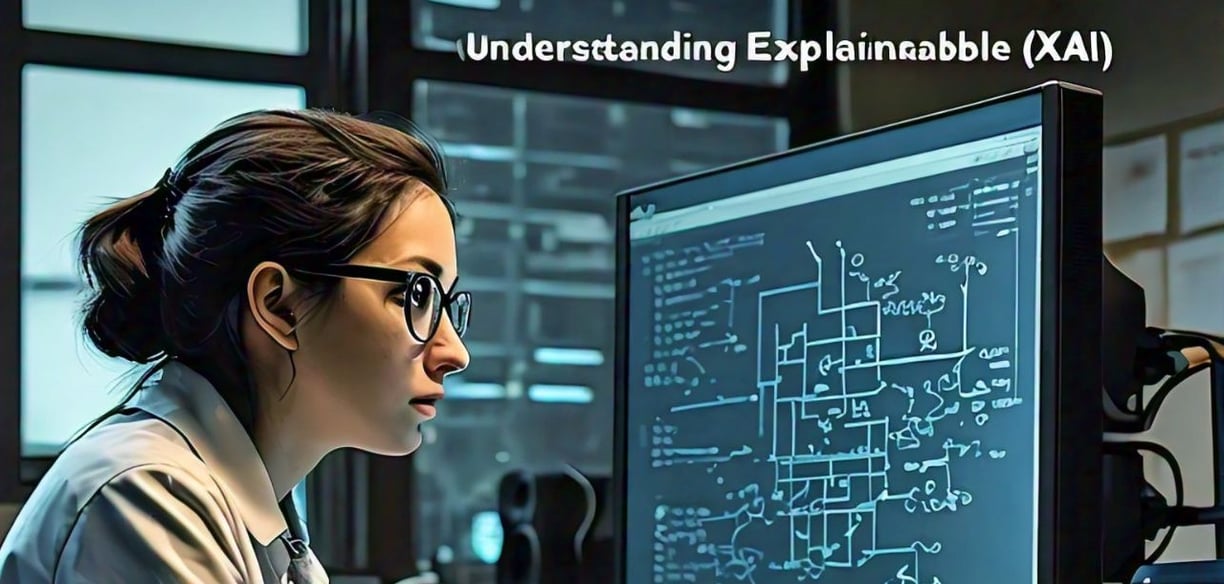
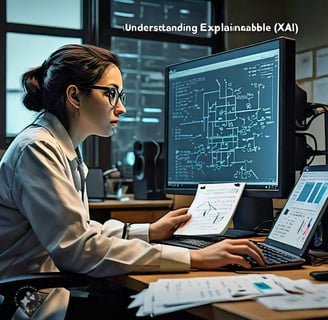
As AI continues to shape various fields such as healthcare and finance, it is increasingly important to ensure transparency and comprehension in AI decision-making. This is where Explainable AI (XAI) becomes essential. XAI involves AI systems that can articulate their decision-making processes in a manner that is comprehensible to humans. In this blog post, we will explore the concept of Explainable AI, its significance, advantages, challenges, and its future role in AI-driven sectors.
What is Explainable AI (XAI)?
Explainable AI (XAI) denotes AI models and systems that deliver clear and easily understandable explanations of how they arrive at their decisions. Historically, numerous AI systems, particularly those using deep learning, have been labeled as “black boxes” due to their lack of transparency regarding their internal processes. XAI seeks to clarify that black box, enabling users and stakeholders to grasp the rationale behind AI predictions and decisions.
Why is Explainable AI Important?
The increasing use of AI in critical fields like healthcare, finance, legal matters, and autonomous vehicles makes trust in AI essential. Transparency in AI systems is critical for various reasons:
Accountability: In regulated sectors such as banking or healthcare, stakeholders must comprehend how AI made a decision to ensure it adheres to legal and ethical standards.
Ethical AI: Explainable AI (XAI) assists in addressing issues related to AI bias, promoting fair and impartial decision-making. By understanding the factors that affect AI decisions, biases can be identified and rectified.
Trust: Users are more inclined to trust and use AI systems when they can grasp how they function. Transparency enhances confidence in AI-driven products and services.
Compliance: With increasing regulations, like the GDPR in Europe that requires accountability and transparency in automated decision-making, XAI supports companies in meeting legal obligations.
Key Benefits of Explainable AI
Improved Decision-Making
XAI offers valuable insights into the reasoning behind AI system predictions, which can enhance decision-making processes. For instance, in the healthcare sector, doctors are more likely to trust an AI diagnosis if they understand the contributing factors. This understanding promotes better teamwork between human professionals and AI.
Bias Detection and Mitigation
Addressing algorithmic bias is one of the primary challenges associated with AI. XAI allows stakeholders to analyze the decision-making processes of AI to spot any biases that may result in unjust outcomes. This is particularly important in areas like hiring algorithms, loan approvals, and legal judgments, where bias can lead to serious repercussions.
Accountability in Regulated Industries
Sectors such as banking, insurance, and healthcare face stringent regulations. XAI helps these sectors stay compliant by providing explanations for decisions, ensuring adherence to ethical and legal requirements.
User Adoption and Trust
When AI decisions can be explained, users are more inclined to trust the technology. This trust is especially essential in AI-driven consumer products like virtual assistants, recommendation systems, and autonomous vehicles. Clear explanations can enhance user confidence and boost adoption rates.
Challenges of Explainable AI
Despite the numerous advantages XAI presents, there are challenges to its effective implementation:
Complexity of Deep Learning Models
Many of the leading AI technologies, especially deep learning models, possess an fundamental complexity. These models often encompass millions of parameters and function in ways that are challenging for humans to grasp. Finding a way to simplify these models for clarity, without compromising accuracy, is a important obstacle.
Balancing Performance and Interpretability
Sometimes, making an AI system more understandable can lead to decreased performance. More detailed models typically provide enhanced predictive accuracy, yet are more difficult to explain. It is necessary to find a middle ground that allows for the creation of models that are both precise and interpretable.
Lack of Standardized Methods
The field of XAI is still developing, and there is currently no universally recognized framework or methodology for making AI systems explainable. Different models may necessitate various approaches to achieving transparency, and the quest for standardized tools for explainability remains a continuous challenge.
Data Privacy and Security
Providing comprehensive explanations for AI decisions may sometimes entail disclosing sensitive information. Bear in mind that XAI systems uphold data privacy and security while ensuring transparency.
Popular Techniques for Explainable AI
Various methods and tools have been created to improve the interpretability of AI models:
SHAP (Shapley Additive Explanations)
SHAP is a widely-used approach for elucidating the outputs of machine learning models. It assigns a score to each feature in the model, reflecting its contribution to the final prediction and helping clarify the significance of individual features.
LIME (Local Interpretable Model-agnostic Explanations)
LIME is another approach used to explain complex models. It generates simpler, interpretable models that mimic the behavior of a more detailed model in specific instances, enabling users to comprehend how predictions are derived.
Counterfactual Explanations
This approach entails providing examples of how the input would need to be altered for a different outcome to occur. For instance, in a loan approval situation, it could illustrate what adjustments in a credit score would lead to loan approval.
Model Transparency Tools
Some AI platforms now offer integrated transparency tools that enable users to visualize decision pathways, examine feature significance, and grasp model behavior without requiring extensive technical expertise.
The Future of Explainable AI (XAI)
As AI becomes increasingly present in everyday life and decision-making processes, the significance of Explainable AI will continue to rise. The outlook for XAI is optimistic, with ongoing advancements in machine learning and model interpretability techniques. Several key trends to monitor include:
Ethical AI Frameworks: As governments and organizations advocate for more ethical AI practices, XAI will be a critical component in AI governance structures.
Regulation Compliance: It is likely that more countries will implement regulations demanding transparency in AI decision-making. XAI will be essential in assisting organizations to meet these regulatory requirements.
Greater AI Adoption: Increased explainability will promote greater trust in AI systems, promoting wider adoption across various sectors, including healthcare, finance, and transportation.
AI and Human Collaboration: As AI systems become more transparent, the collaboration between humans and AI will enhance. Professionals from diverse fields will be able to work alongside AI more effectively, using it for data-driven insights and explanations.
Explore
Motivating material related to health, technology, finance, and lifestyle in everyday life.
Connect
Discover
info@webslike.com
© 2024. All rights reserved.